This curse is separated in 6 main sessions.
Each of them comprises of 3,5 hour and contains analogy-based mathematical concepts, discussions, and practical interactive exercises.
Despite including mathematical foundations, definitions and concepts,
the main focus of this course is to foster a deeper understanding on how machine learning model are capable of learning various structures.
Sessions can require preparations/ pre-class readings and/or include a post-class exercise.
Those exercises will help you, to get a better start with your final projects.
In the final session, we invite a guest lecturer for further insight into current topics in the field.
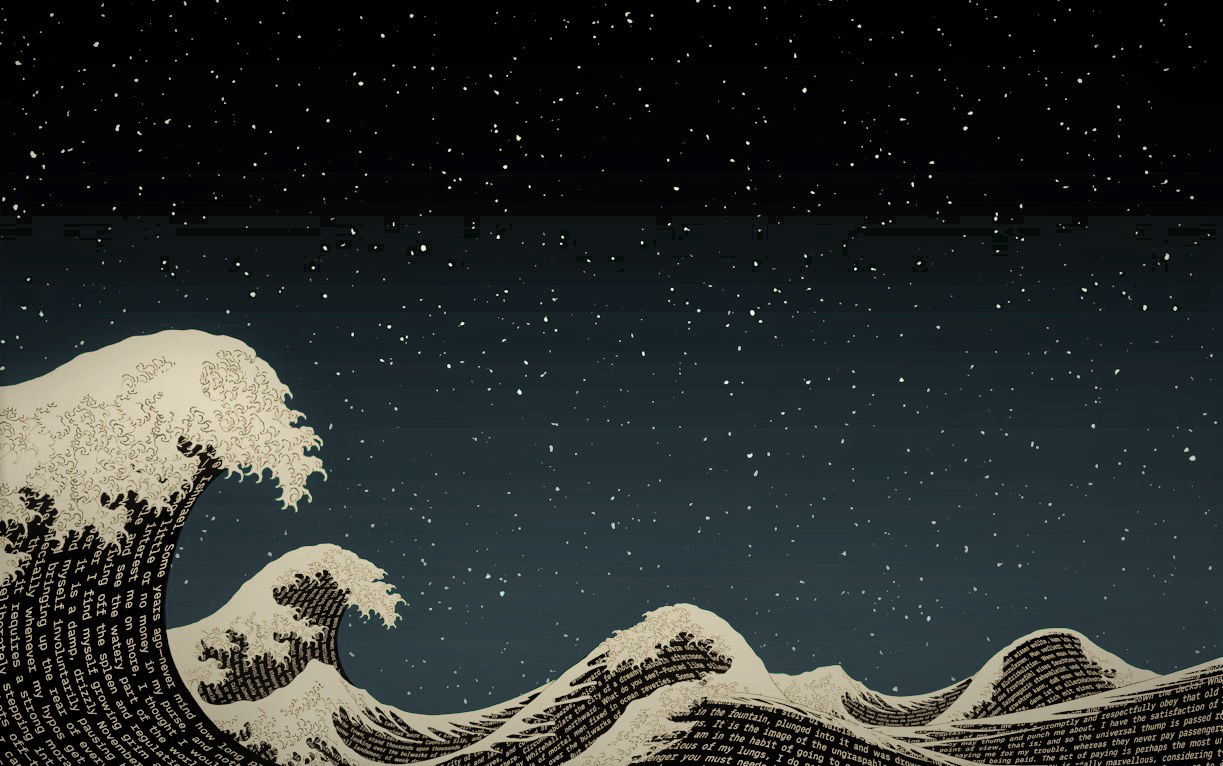
01:Intro & Overview
What is Artificial Intelligence?
Supervised & Unsupervised Learning
Data, Features, Decision Boundaries
View more
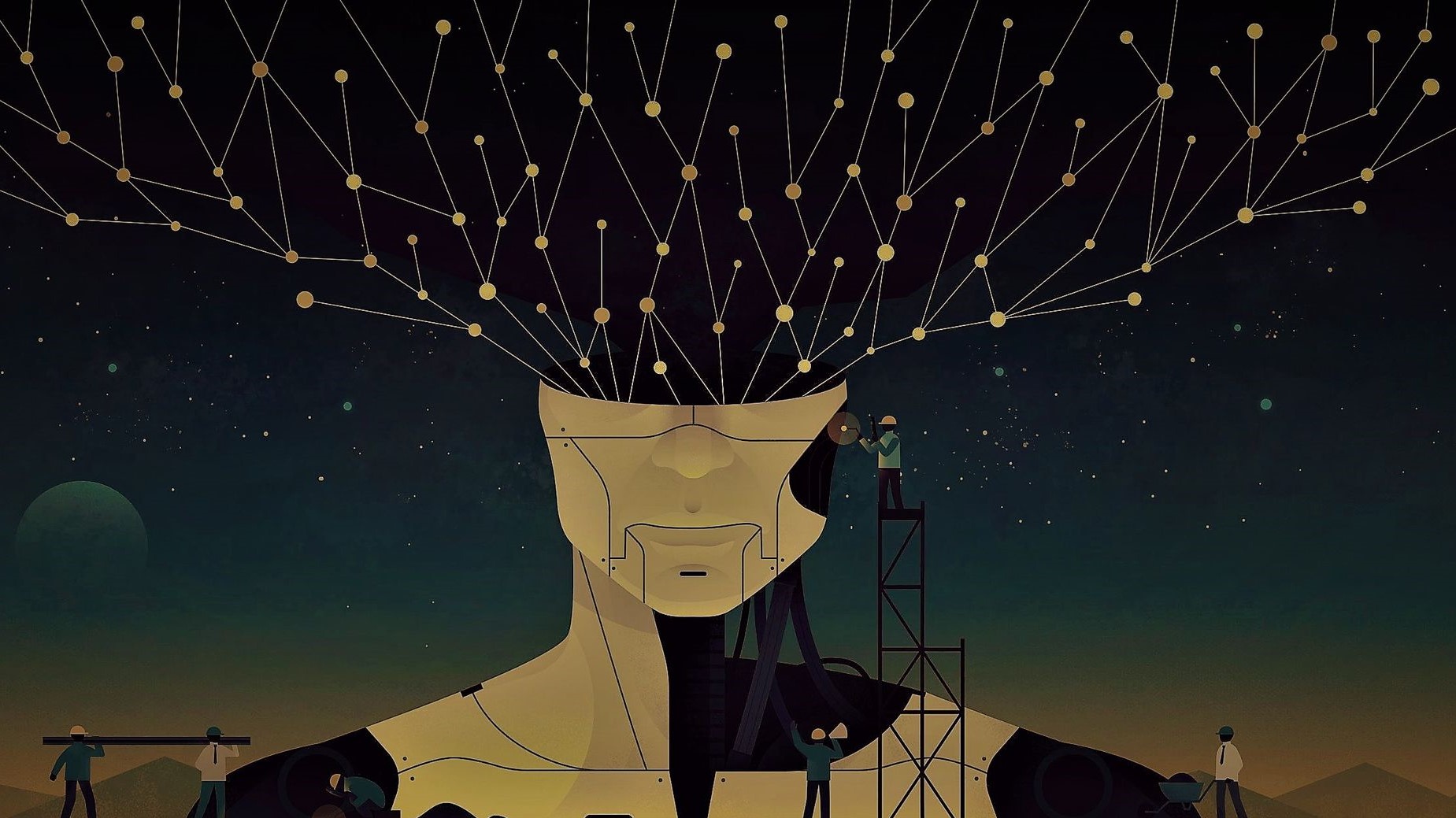
02:INT(R)O Neural Networks
The Biological Neuron
The Perceptron & Non-Linear Decisions
Geons, Bigrams & Feature Detection in Images
View more
View more
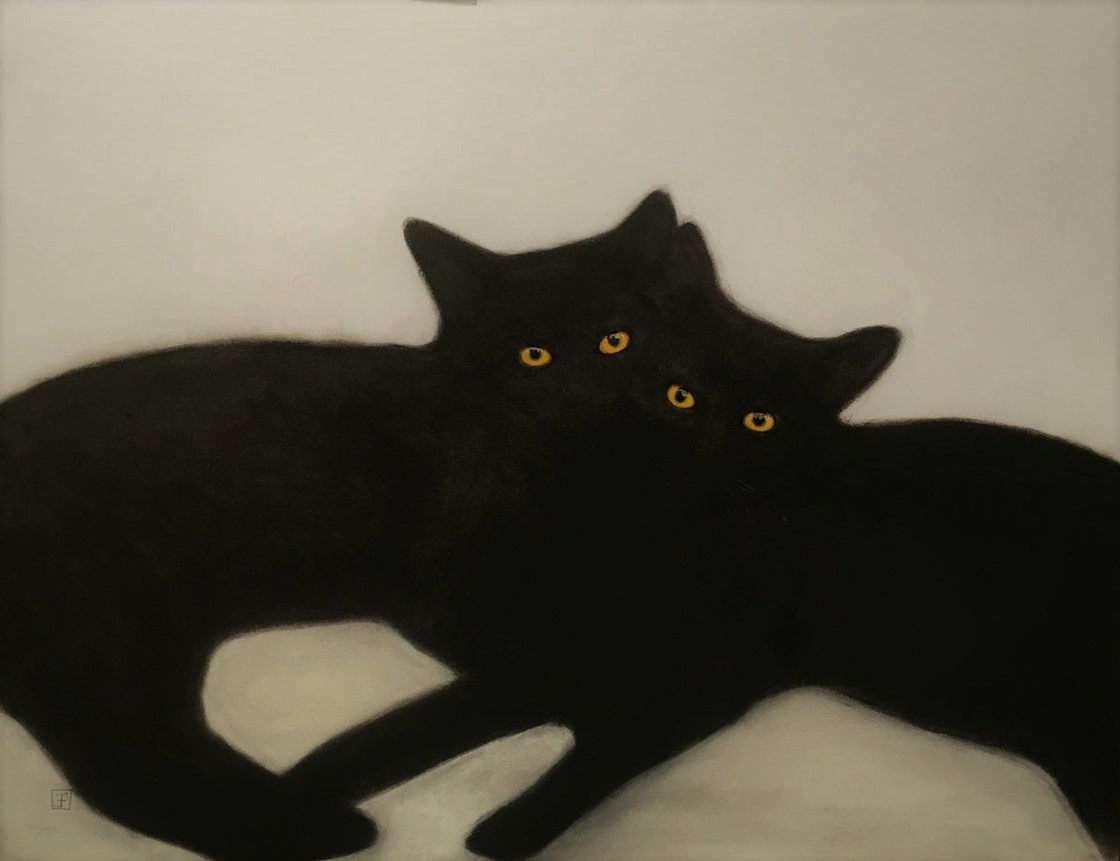
03:About Data Cats & Dogs
BIAS from Data
Vector, Matrix & Tensor
How does a Convolutional NN work?
View more
View more
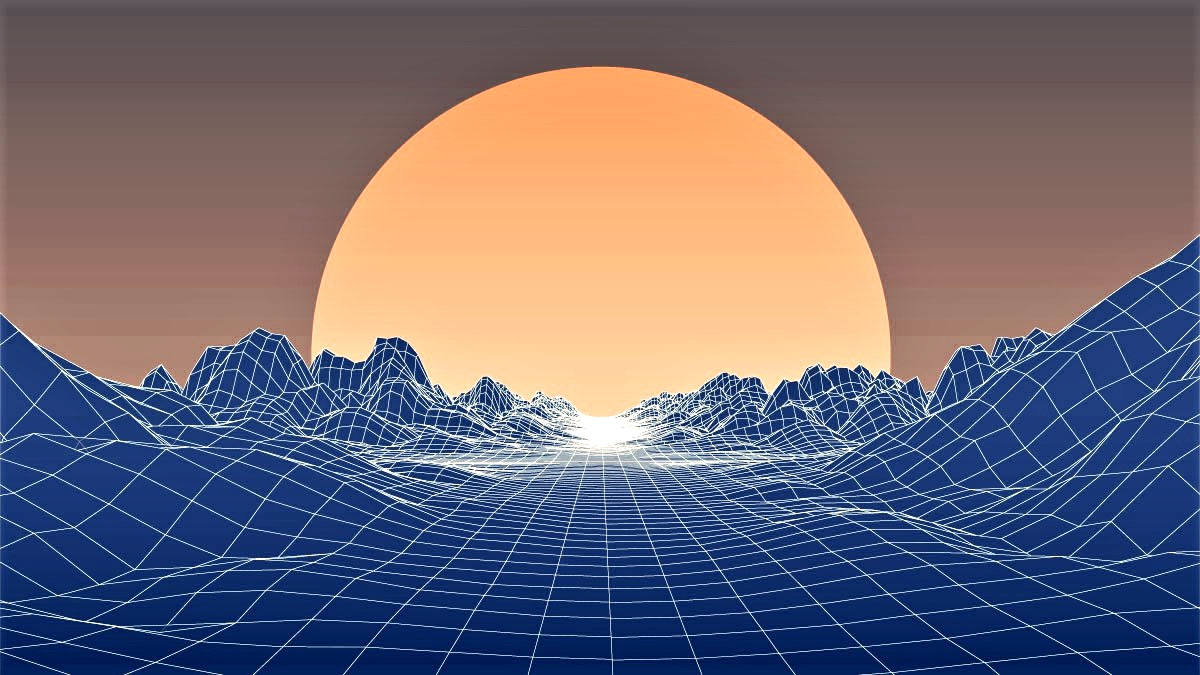
04:Optimization
Objective Functions
Optimizers and why they are so powerful
Numerical Optimization, local vs global minima
View more
View more
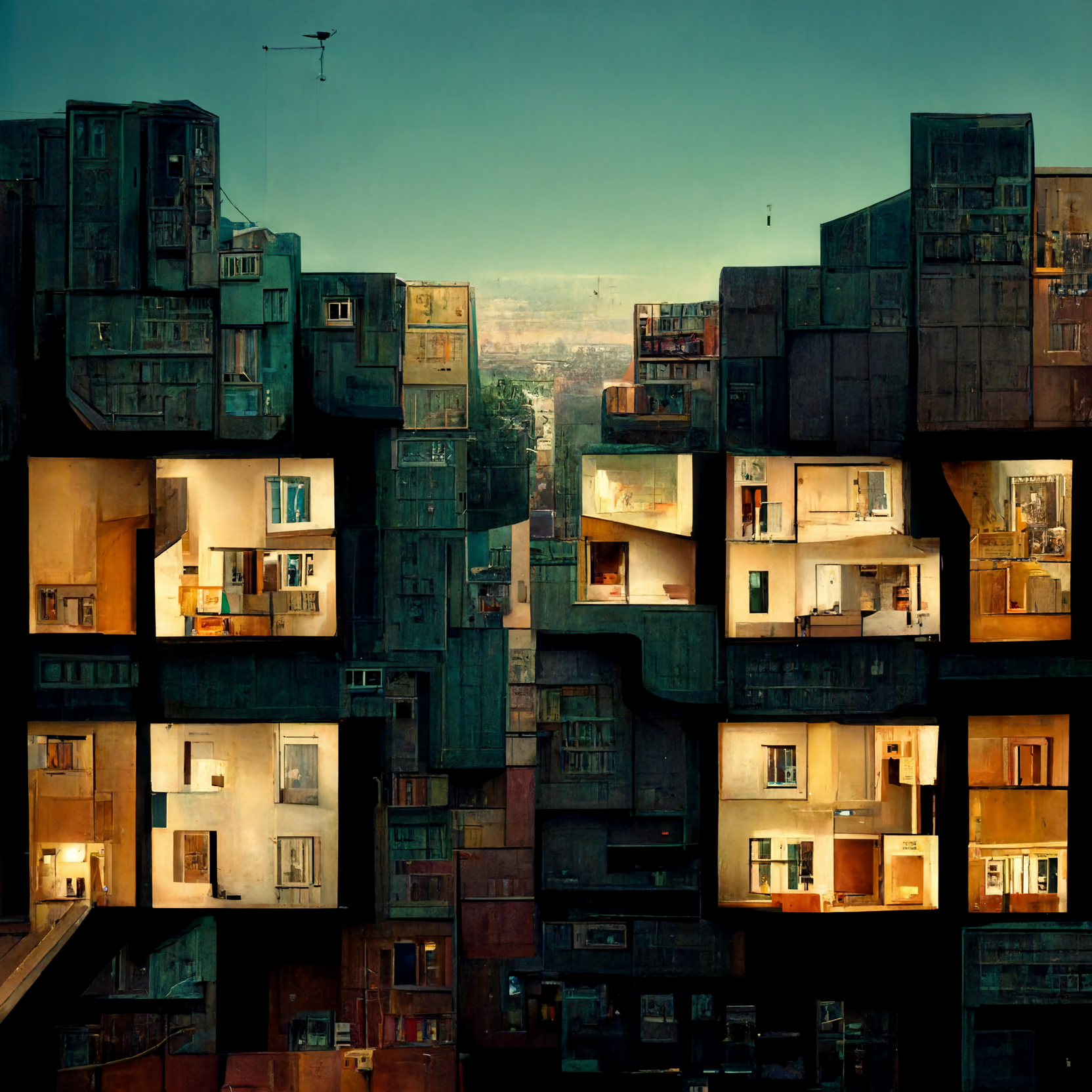
05:Architectures: knot by knot
Architecture by Layers, Design and Modification
Feed-Forward, Convolutional and LSTM layers
(Variational) AutoEncoder, Siamese Networks and Generative Adversarial Networks
View more
View more
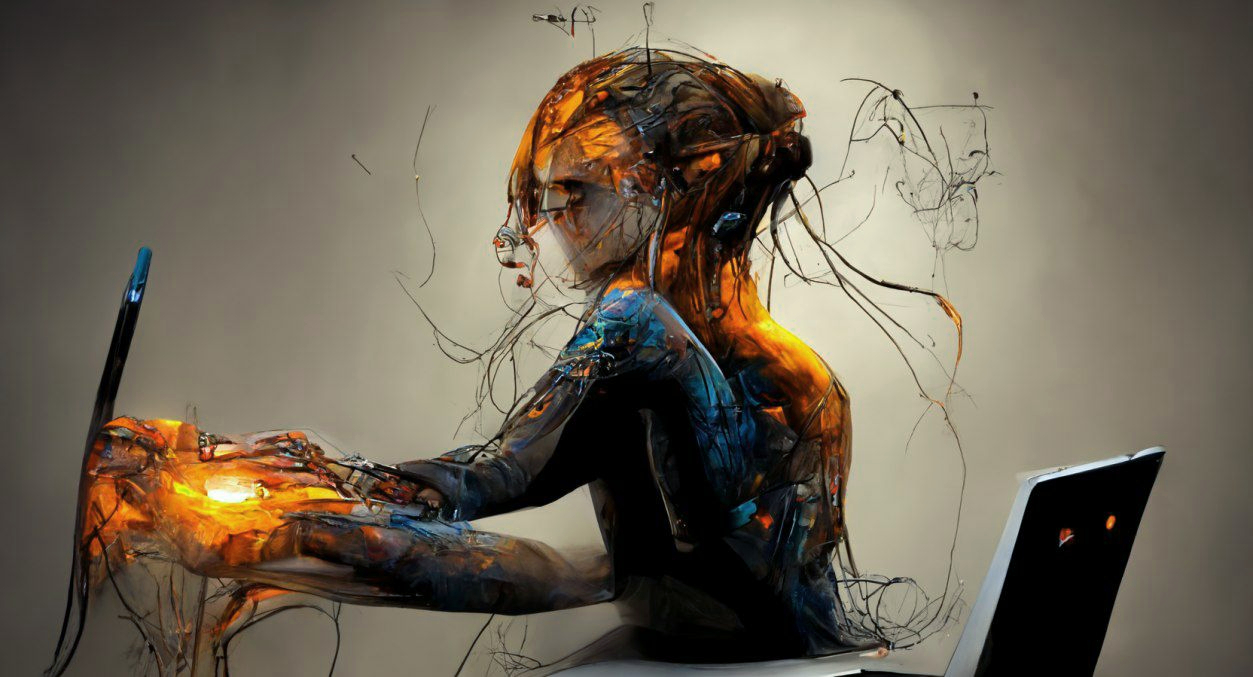
06:Critical
NN studies
Decomposing Information
What can an ANN not learn??
Generalization & catastrophic forgetting
A bitter lesson: human knowledge or comp. power?
View more
View more
Guest lecture:
The guest lecture in the winter term 22/23 will be held by Lukas Bonauer.
He will talk about his experiences at DeepMind
and address topics such as Natural Language Processing (NLP), Artificial General Intelligence (AGI), and the resulting safety issues and impact on society.